30th May, 2023
Dr. Furqan Afzal: On Creating AI tools to study Neuroscience
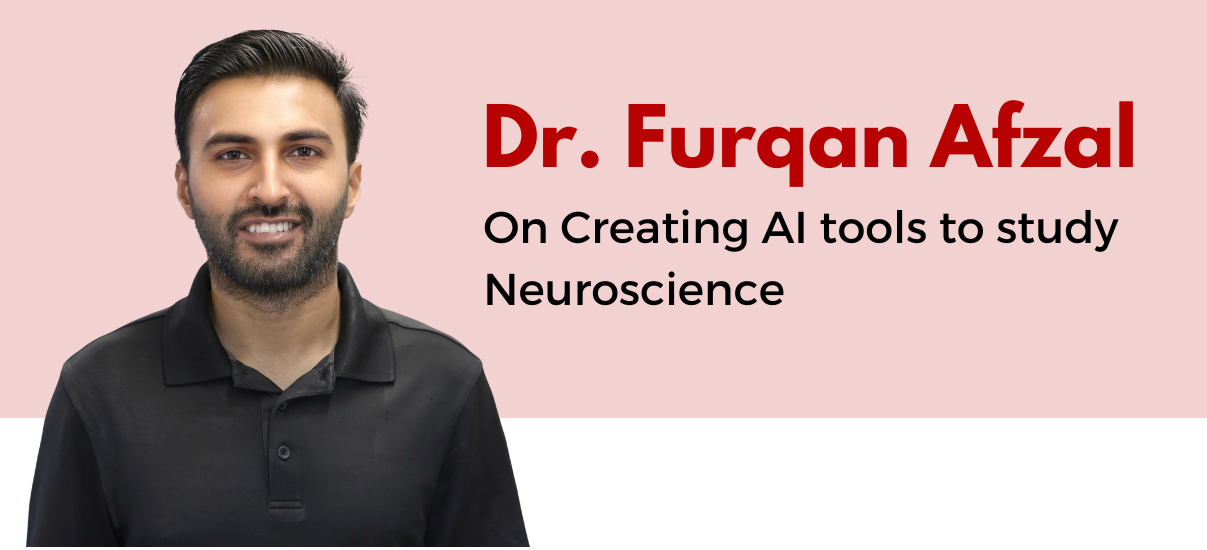
Dr. Muhammad Furqan Afzal recently completed his PhD in Computational Neuroscience at the Icahn School of Medicine at Mount Sinai in the USA, where he focused on the intersection of neuroscience and AI, and developed AI tools for extracting insights from brain activity data. He holds a Master of Science degree in Computational Neuroscience from the University of Cincinnati, and obtained his Bachelor of Science degree in Electrical Engineering from LUMS SSE in 2014.
Dr. Afzal has also worked as a Research Scientist at Stanford University, where he utilized AI and signal processing techniques to identify neural biomarkers associated with symptoms in Parkinson’s disease. He has also gained experience in neurotech companies and startups, contributing to the development of AI-based tools for brain-computer interfaces (BCI). His research interests encompass various areas including neuroscience, artificial intelligence and its applications in healthcare.
How does Data Science and Neuroscience converge? And how is it beneficial for the world?
We are gathering vast amounts of data from neuroscience experiments conducted on both humans and animals. These experiments are conducted with the aim of investigating different processes such as decision making, memory, and motor control. Additionally, we conduct experiments to explore brain-based or other biological markers associated with various neurological or psychiatric conditions such as Parkinson’s disease, epilepsy, or depression. This is where the importance of data science and artificial intelligence (AI) emerges. Given the overwhelming volume of data, data science and AI enable us to extract valuable insights from the raw data concerning the brain mechanisms underlying different normal processes and neurological conditions.
In the field of computational neuroscience, we also frequently construct computational models to simulate various brain functions, and data science proves to be useful in building and exploring those models. These models, in turn, can generate testable hypotheses for further experiments.
What was your PhD thesis about and what were your findings?
During my doctoral studies, my research focused on developing innovative machine learning and AI techniques that proved valuable in extracting insights from neuroscience data, as mentioned earlier. Broadly speaking, my work encompassed three distinct neurological conditions: Parkinson’s disease, depression, and epilepsy. In these domains, I employed data science and AI methodologies to identify neural or brain-based biomarkers associated with various symptoms observed in these conditions, such as tremors in Parkinson’s disease or seizures in epilepsy.
Specifically, within the scope of my PhD, I created AI tools designed for time-series classification of brain data, including EEGs (electroencephalograms) and local field potentials. Utilizing these tools, I successfully detected changes in brain states among patients undergoing treatment for major depression when they had improvements in their symptoms. Consequently, these AI tools can be utilized to identify and monitor the progression or regression of symptoms in patients by analyzing their brain data.
I am actively engaged in the development of biologically inspired AI tools that aim to simulate the functioning of the brain more closely. The underlying concept is that by drawing inspiration from the intricate workings of our own brains, these tools have the potential to exhibit enhanced performance capabilities.
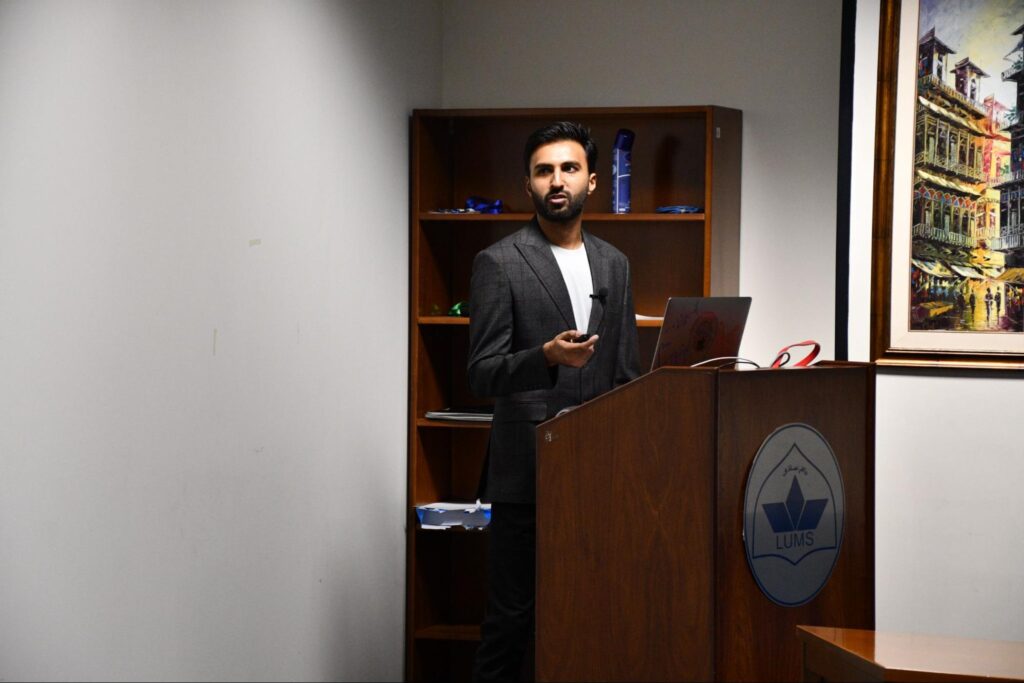
What is the most interesting model / tool / computational structure you have worked with thus far? And what has been the most surprising insight it has generated?
Our primary focus lies in identifying and addressing the actual problems that need to be solved rather than solely emphasizing on the tools themselves. Our research encompasses diverse problem domains, such as the discovery of brain-based biomarkers for diseases using time-series data or the development of innovative biologically inspired AI tools. Once we have identified the problems at hand, we can then consider the most suitable tools for solving them. The fields of computational neuroscience and AI have yielded an array of remarkable tools that assist researchers in efficiently tackling their problems and guiding them towards effective solutions.
Personally, I have extensively worked with deep recurrent neural networks in neuroscience research and have found them to be highly beneficial. These networks are loosely inspired by the feedback connections observed among neurons in our brains. With their inherent memory capabilities, these networks serve as valuable testbeds for exploratory investigations, allowing us to generate insights and formulate testable predictions. Moreover, they prove to be effective tools for analyzing various types of time-series data that we collect during our research.
What are the biggest areas of opportunity in the Computational Neuroscience field?
In the field of computational neuroscience, there are several significant areas of opportunities that hold great potential for advancement and impact. Some of the biggest areas or subdomains include brain-machine Interfaces (BMIs) where we work on direct communication and interaction between the brains and external devices. This includes the development of neuroprosthetics and technologies that restore or enhance sensory, motor, or cognitive functions.
We also have neural circuit modeling which involves creating detailed computational models that simulate real neural networks, allowing us to investigate the mechanisms underlying various brain functions and behaviors.
Neural data analysis allows us to analyze and integrate large-scale neural data from diverse sources, such as electrophysiological recordings, imaging modalities, and genetic information. This involves the development of algorithms and statistical methods to extract meaningful insights from complex and high-dimensional datasets.
We can use computational approaches to gain insights into the underlying mechanisms and causes of neurological and psychiatric disorders. This includes identifying biomarkers, studying disease progression, and developing computational models that can aid in diagnosis, treatment, and personalized medicine.
We can develop closed-loop systems for adaptive interventions that dynamically interact with the brains in real-time, adjusting therapy based on ongoing neural activity. Thisincludes closed-loop neuromodulation techniques for therapeutic purposes and adaptive training paradigms for neurorehabilitation.
These areas of opportunity highlight the immense potential for computational neuroscience to contribute to our understanding of the brain and its disorders, advance medical interventions, and drive innovation.
Can you tell us about a phenomenon that is in its infant stage as of yet but has the potential to transform the way we perceive neuroscience today?
One of the most influential areas of research in neuroscience today is the study of brain connectivity and network dynamics. This subdomain focuses on understanding how different regions of the brain are connected and how these connections contribute to brain function and behavior. The advancements in neuroimaging techniques, such as functional magnetic resonance imaging (fMRI) and diffusion tensor imaging (DTI), have significantly contributed to this area of research. Researchers are investigating the complex patterns of connectivity within the brain, known as the connectome, and how these connections change during development, learning, and in various neurological and psychiatric disorders. This research has revealed important insights into the organization and communication of brain networks, highlighting the importance of both local and long- range connections in information processing.
Furthermore, the study of network dynamics explores how brain networks dynamically change and adapt in response to cognitive tasks, environmental stimuli, and disease states. This research focuses on understanding how brain networks reconfigure themselves to support different cognitive processes and how disruptions in network dynamics may contribute to neurological and psychiatric conditions. It has also contributed to the development of new diagnostic and therapeutic approaches, such as using brain connectivity measures as biomarkers for disease detection and developing targeted neuromodulation techniques.
It is intriguing to note that we have the ability to construct computational/AI models, such as those utilizing recurrent neural networks, that incorporate artificial units mirroring the firing characteristics of actual neurons observed in experiments involving animals or humans. By employing these artificial networks, we can investigate and explore how the dynamics of the networks are altered during disease conditions and in the presence of external stimuli. This approach allows us to generate valuable insights and formulate testable hypotheses regarding the changes in network dynamics under various circumstances.
Overall, the study of brain connectivity and network dynamics is a highly influential and rapidly evolving area of research in neuroscience, offering profound implications for our understanding of the brain and its complex functions.
What is your advice for those interested in becoming a neuroscientist?
To excel as a neuroscientist, it is crucial to embrace interdisciplinary learning. Neuroscience has evolved into a field that draws from diverse disciplines such as biology, psychology, computer science, physics, and mathematics. Having a broad knowledge base will help you understand the intricate workings of the brain.
Additionally, proficiency in data science and AI is increasingly becoming important in neuroscience. The field generates vast amounts of data, and data science techniques are vital for extracting meaningful insights from complex datasets. Familiarize yourself with data analysis methods, statistical approaches, and programming languages commonly used in data science.
Staying up-to-date with the latest literature is crucial in such a rapidly evolving field. Develop a habit of reading scientific papers regularly to stay informed about new discoveries, breakthroughs, and emerging trends in neuroscience. This will help you identify research gaps and opportunities for exploration.
Collaboration and networking are fundamental to success in neuroscience. Embrace collaborations with researchers from different disciplines to gain diverse perspectives and foster innovative projects. Attend conferences, seminars, and workshops to network with fellow neuroscientists, exchange ideas, and establish professional connections.
Strong critical thinking and problem-solving skills are essential in neuroscience research. The field presents us with complex questions and challenges that require the cultivation of these skills to tackle them effectively.
Becoming a successful neuroscientist requires dedication, continuous learning, and a passion for unraveling the mysteries of the brain. By embracing interdisciplinary knowledge, integrating data science and AI skills, engaging in research, and fostering effective communication, you should be on your path to becoming a good neuroscientist.