DALL-E, an innovative AI model developed by OpenAI, is revolutionizing the way we create images from text descriptions. This cutting-edge technology combines language and visual processing, allowing users to describe an image in words and have DALL-E generate it, even if it’s a concept that doesn’t exist in reality.
Imagine being able to describe a fantastical creature, a futuristic cityscape, or a whimsical scene, and having DALL-E bring it to life in visual form. This unique capability opens up endless possibilities for artists, designers, educators, and communicators alike.
One of the most exciting aspects of DALL-E is its ability to bridge the gap between language and visual art. By understanding and interpreting textual descriptions, DALL-E can create images that capture the essence of the words, offering a new way to express and explore ideas visually.
DALL-E’s impact goes beyond just creating images; it has the potential to transform industries such as advertising, design, and storytelling. With DALL-E, the only limit is your imagination.
When was DALL-E launched?
DALL-E, which made its debut in January 2021, is a variant of OpenAI’s GPT-3, a groundbreaking language-processing model.
Additionally, the name “DALL-E” combines references to the surrealist artist Salvador Dalí and Pixar’s animated robot Wall-E, showcasing its whimsical nature. Its successor, DALL-E 2, launched in April 2022, focuses on generating more lifelike images at higher resolutions.
What is the difference between GPT-3 and DALL-E?
Unlike GPT-3, which deals solely with text, DALL-E adds a visual dimension by generating images from textual descriptions. This is achieved through a transformer neural network, a type of AI architecture that GPT-3 also uses, but trained specifically for image creation.
Both GPT-3 and DALL-E learn through unsupervised learning, where they analyze large datasets of text-image pairs. This process involves optimizing the model’s parameters through backpropagation and stochastic gradient descent, essentially fine-tuning the model based on its performance.
DALL-E learns to associate textual descriptions with visual elements by recognizing patterns and relationships in the data. For example, when repeatedly exposed to images of dogs alongside the word “dog,” it learns to connect the text with the visual concept.
This ability extends to more complex associations, enabling DALL-E to generate images based on detailed and sometimes surreal descriptions.
With enough training, DALL-E can create original images that match given textual descriptions, showcasing its creative potential. Its applications span from generating unique artworks to aiding visual communication in fields like education and design.
For example, DALL-E can help educators create visual aids for abstract concepts, demonstrating its versatility and potential impact across various industries.
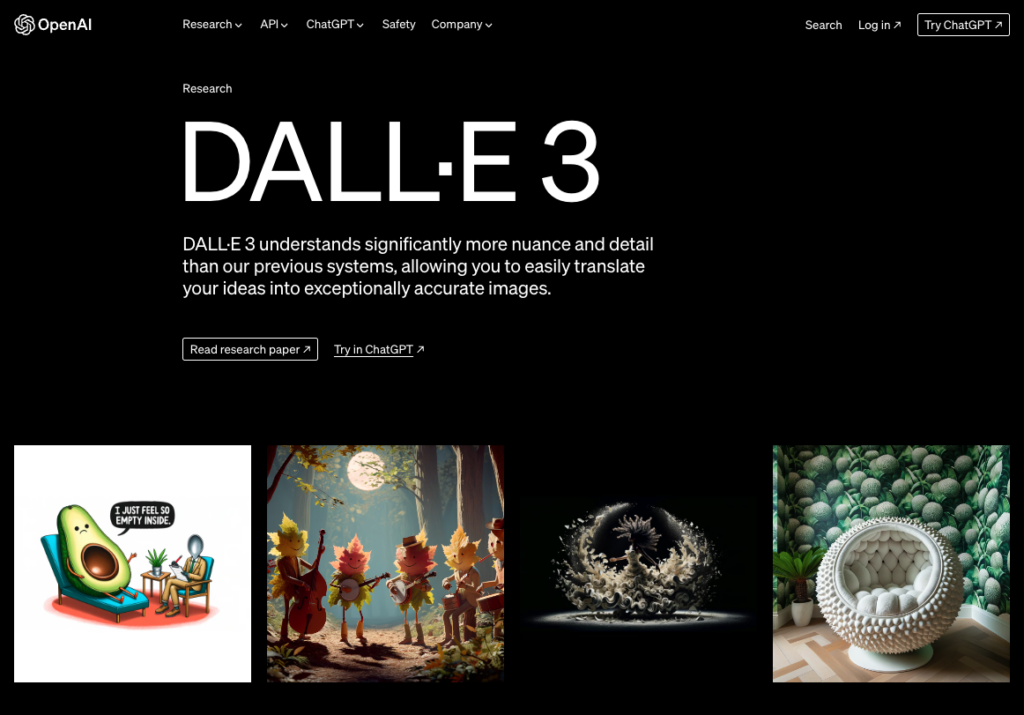
What are the real-world use cases of DALL-E?
Here are some real-world examples of how DALL-E can be used:
- Education: DALL-E can help educators create visual aids for abstract concepts. For example, it could generate images to illustrate complex scientific theories or historical events, making learning more engaging and accessible for students.
- Design: Designers can use DALL-E to generate custom artwork based on specific descriptions. For instance, they could describe a scene or concept, and DALL-E could create an initial draft or concept art, speeding up the design process.
- Marketing: In marketing, DALL-E could be used to create unique images for advertising campaigns. Marketers could input descriptions of products, target audiences, and desired moods, and DALL-E could generate custom graphics that align with the campaign’s goals.
- Storytelling: Authors and storytellers can use DALL-E to create visual representations of their narratives. For example, they could describe a character or setting, and DALL-E could generate an image to accompany the story, enhancing the reader’s experience.
- Fashion: In the fashion industry, DALL-E could be used to create digital designs for clothing and accessories. Designers could describe their vision for a garment, and DALL-E could generate a visual representation, helping designers visualize their concepts before creating physical prototypes.
- Interior Design: DALL-E could assist interior designers in visualizing spaces. Designers could describe a room’s layout, color scheme, and furnishings, and DALL-E could generate images to help them visualize the final design.
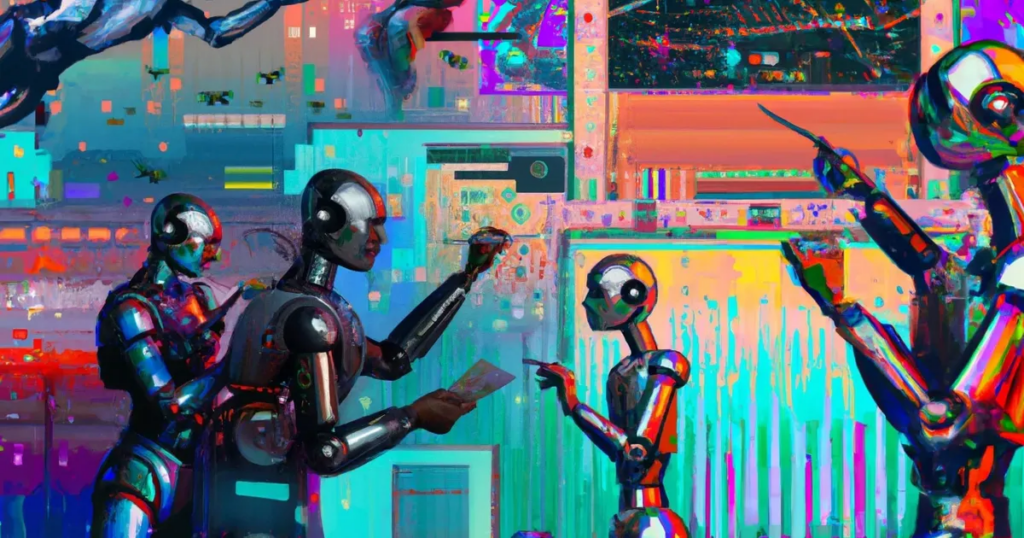
What are the pros and cons of DALL-E?
Pros | Cons |
Quickly generates images from text, saving time and resources compared to traditional methods. | Output isn’t always predictable or fully controllable, challenging for precision applications. |
Visualizes complex concepts, expanding creative possibilities. | Concerns over copyright infringement if images resemble copyrighted works too closely. |
Creates highly tailored visuals for specific needs. | Without proper moderation, DALL-E could generate inappropriate or harmful content. |
Democratizes custom graphic design for small businesses and creators. | Automation could displace jobs in graphic design, though new roles in AI management may emerge. |
What are the DALl-E alternatives?
While DALL-E remains a popular choice for AI image generation, there are other alternatives gaining recognition. Two notable ones are Midjourney and Stable Diffusion:
- Midjourney: Developed by an independent San Francisco-based research lab, Midjourney offers high-quality, detailed image generation. It’s currently in open beta and accessible through Discord, but requires payment for image generation.
- Stable Diffusion: This open-source tool was initially trained on 2.3 billion images by researchers from the CompVis Group at Ludwig Maximilian University of Munich, StabilityAI, and RunwayML. It has a growing community and offers both free and paid versions.
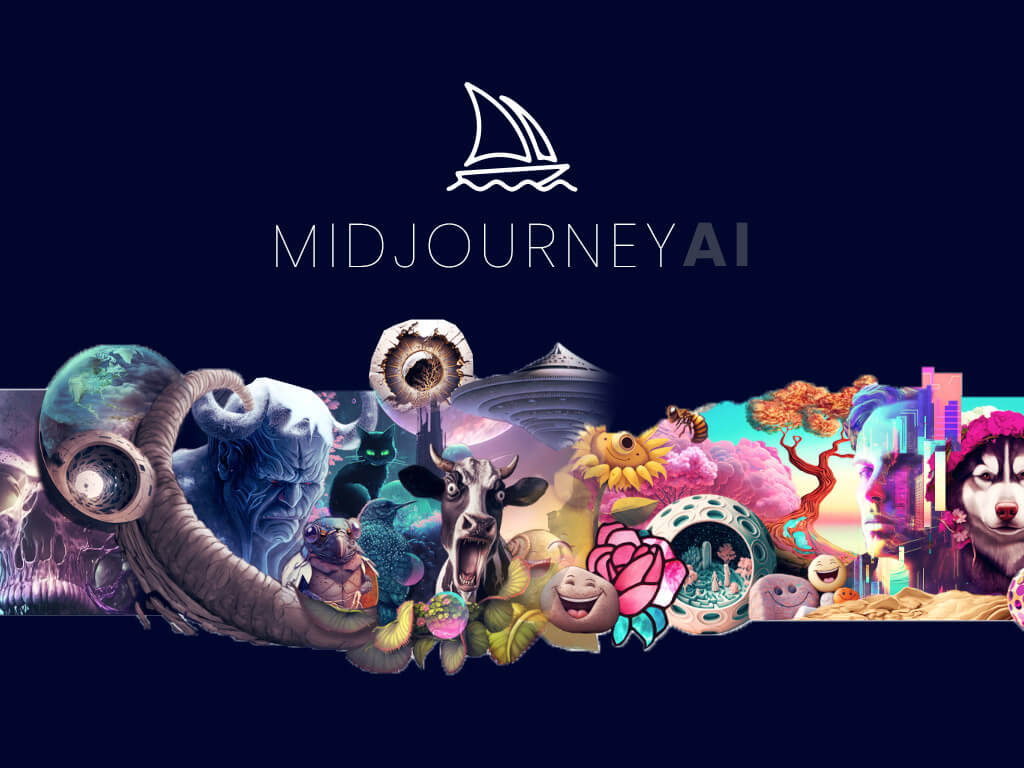
How to use DALL-E effectively?
For optimal use of DALL-E, consider the following suggestions:
- Provide Specific Details: Offer clear and detailed descriptions of your desired image.
- Experiment: Try various text descriptions to explore DALL-E’s capabilities.
- Use Precise Language: Employ clear and exact language in your descriptions for the best outcomes.
- Request Image Quality: Specify “highly detailed” or “high-quality” to ensure the images meet your standards.
- Explore Styling Options: Experiment with different styles, lighting, effects, and backgrounds for unique images.
- Engage with the Community: Join communities to share experiences and learn from others using DALL-E.
Conclusion
Generative AI models have evolved into graphic design tools, allowing users to replace backgrounds, add objects, make edits, and manipulate images using just a selection tool and a prompt.
This advancement eliminates the need to rely on graphic designers for tasks like creating logos or designing posts. These new tools, based on DALL-E, are revolutionizing the creator landscape.