Have you ever looked at a very cool picture of a star or a galaxy and wondered how astronomers capture such images of celestial objects millions and millions of miles away?
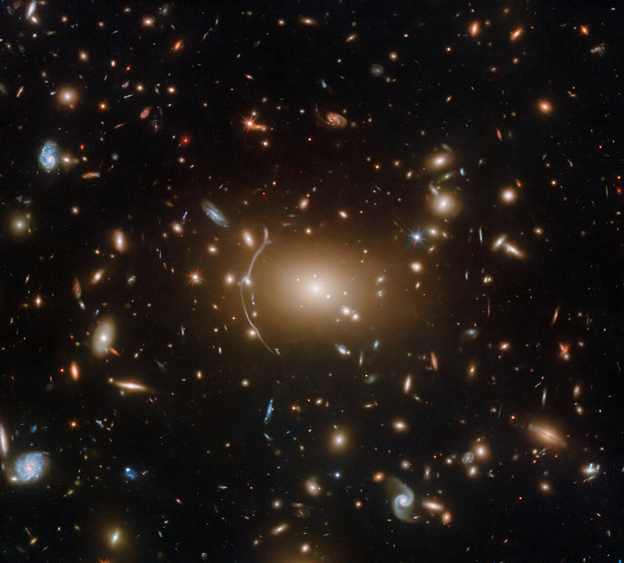
Galaxy Cluster Abell 611, located roughly 3.2 Billion Light Years from Earth – Hubble Telescope
Well, data science plays a crucial role in helping space scientists image distant stars and galaxies. In recent years, astrophysics and data science have become closely intertwined. Gone are the days of old when astronomers would meticulously sift through a multitude of stellar spectra by eye and categorise stars. Nowadays you would employ a machine learning code with all known spectral types already fed into it. Of course, every now and then one might still have a closer look at a peculiar star or two. Classifying galaxies based on their morphology is another avenue where machine learning has been used to disentangle galaxies that are morphologically ambiguous or in simple words, just plain weird. This, of course, only being possible once the code has been trained using galaxies of known morphological types.
The vast amount of data that is collected from space telescopes can be overwhelming, and data science provides the tools and techniques needed to extract useful information from this data. Raw data collected by space telescopes like Hubble, Chandra, Spitzer, etc., are often noisy, blurred, and distorted. Data scientists use advanced image processing techniques to clean up the data, remove noise, and enhance the resolution. This helps in producing clear and sharp images of distant stars and galaxies.
One important application of data science in space exploration is in image processing. Space telescopes capture images of distant stars and galaxies that are often blurred or noisy. Data science techniques such as image processing algorithms can be used to enhance these images, removing noise, and improving resolution. This allows astronomers to see more clearly and extract more information from the images. Another application of data science in space exploration is data analysis. Space telescopes collect massive amounts of data, and data science provides the tools needed to analyse this data and extract meaningful insights. Data science techniques such as machine learning can be used to identify patterns in the data, helping astronomers to classify stars and galaxies and make predictions about their behaviour.
A key tool that has become the bread and butter of astrophysicists from across various subfields is computational modelling. What makes astrophysics unique is that many times the objects being studied are distant and not accessible unless one is studying a celestial body in the Solar System. Say someone wanted to understand the interior of a neutron star or a dust clump falling onto a black hole. Well, what does one do? Construct a model you see! Create a numerical code using known physical laws, assign some parameters “realistic” values that you would expect and then solve these equations on a user-defined grid iteratively to see how the particular astrophysical scenario evolves in time. So, for instance, one could model the effects of the solar wind on Earth’s atmosphere. Or perturbations occurring inside a blackhole accretion disk because of a nearby star. The possibilities are limitless. Stellar codes are a common commodity in astrophysics the results of these codes are often at times directly compared with observations. For example, one can model the interior of a pulsating star and check if the pulsation period agrees with what is observed. As computing power continues to increase so does the resolution and scope of these numerical codes. Thus, with time we expect astrophysical codes to become more accurate and robust. Lastly, let us not forget that historically for the most part astronomers have been taking images of the night sky. That tradition continues albeit with a lot more sophistication than what was previously required. For instance, the famous M87 blackhole image was the result of a technique known as interferometry. For those of us in the field, constructing images with interferometry can be a handful. Thus, a great degree of ingenuity is required in order to solve the problem. Over the last few decades, astronomers have developed a plethora of image reconstruction algorithms that with time have yielded better and better images. Essentially these algorithms tend to fill in the “spaces” left out by observations in order to construct a complete image. This filling in of course relies on the underlying mechanism that the algorithm uses, for example, MCMC or Baysian inference. Some of the more cutting-edge softwares have started employing neural networks as well, and this is expected to revolutionize interferometric image reconstruction in the near future.